INBOX INSIGHTS: Marketing Mix Modeling (4/5) :: View in browser
April 2023 One Click Poll
Please click/tap on just one answer – this is our monthly survey to see how we’re doing, so please do take it each month!
How likely are you to recommend Trust Insights as a consulting firm to someone in the next 90 days?
We use this information to measure how effective our marketing is. There’s no form to fill out – tapping your answer is literally all there is to it, and then you get to see Katie’s dog.
Getting Started with a Marketing Mix Model [title]
I said to Chris earlier, “I feel like everyone is suddenly talking about running a Marketing Mix Model. It’s not new, right? Why is it suddenly such a popular topic?”
He told me one of the big reasons is data privacy. Attribution models, which are similar, but not the same, will struggle to be accurate and valuable as data privacy continues to restrict the data they can access.
What does that mean?
Have you noticed in your Google Analytics account there is more “unassigned” and “direct” traffic, even if you’re being diligent with your UTM tagging? This is because of the data privacy regulations that are protecting the users visiting your website. This means that attribution models, like the ones you can get from Google Analytics, will be less and less effective in telling you which channels are working.
As a quick refresher: an attribution model tells you what gets credit for a user taking a specific action. Most commonly in marketing, we use attribution reporting to understand what actions led to certain conversions, like opening an email led to a form fill. A marketing mix model is a large regression model. A regression model is trying to understand the relationship between variables, such as weather patterns and revenue. You can still try to understand what actions lead to conversions, but a marketing mix model allows you to introduce more data. The analysis can then tell you the relationship between the variables. For example, you bring in more sales when it’s sunny outside because you’re a brick-and-mortar shop and nice weather means more foot traffic.
Here’s a real example from this morning. Chris and I were working through the monthly reports. We run attribution reporting for ourselves and our clients. Because I know our data so well, I flagged what looked to be some inaccuracies. Fast forward two hours later and as we’re peeling back the layers, we’re realizing that there are major discrepancies between what we get from our own website, and what Google Analytics tells us. Further, there are discrepancies between the Data API and the Google Analytics interface. I shouldn’t have to mention this but also know that there are HUGE discrepancies between Universal Analytics and GA4. We know what the metric should be, and yet none of our data sources match. The issue here is that we’re restricted to one data set for the attribution model. With a marketing mix model, we can look outside the problematic data for something more accurate.
Before we can get into the analysis, we have to take inventory. The example above demonstrates why you need to do requirements gathering and have strong data governance before running a marketing mix model. If you don’t have a good handle on what your data should tell you, a complex analysis won’t help. Let’s do a quick run-through of the 5Ps so we can understand what we’re working with. As a refresher, the 5Ps are Purpose, People, Process, Platform, and Performance.
Purpose
This is where you’ll state the reason you want to run a marketing mix model. The best way to organize your throughs is with a user story.
As a [persona], I [want to], so [that].
A #protip is that the user story tells you what the other Ps are. “Persona,” tells you the people, “want to,” tells you the process and platform, and “that,” tells you the performance. Here’s what mine looks like:
As a CEO, I want to understand which of my digital marketing efforts are resulting in sales, so that I can prioritize budget and resources.
In this statement, I have a lot of information. Let’s keep breaking it down.
People
I stated that I wanted to understand the data, so I’m the first person involved. Knowing that I am not solely responsible for Trust Insights data collection and analysis, I can reasonably assume that Chris will be involved as well. Since we’re also talking about sales information, John will need to participate.
Process
I stated that my purpose was to understand my digital marketing efforts and sales. In terms of process, this statement tells me that I need to do a couple of things. I need to know how that data is being collected, the frequency, and the format. This is where I need to have data governance in place so that the data collection processes aren’t what holds up running a marketing mix model. Once I identify which systems I need to extract data from (in the next step), I can circle back up to the processes, making sure that I can export the data needed. If I can’t, I’ll need to develop new processes and work them into the overall plan. I will also need to create a process to clean and normalize the data once it’s extracted so that I can analyze data from different sources. If I were getting the user story from a stakeholder, I would probably push back and ask for a more specific timeframe as well. This is where you will likely spend most of your time, between process and platform. With a marketing mix model you can have multiple data sources that won’t necessarily export into the same exact format, meaning you have to build process to combine your data for analysis. And the more data you want to use from different platforms, the more process you’ll need to develop. Especially if you want to rerun the marketing mix model again and again.
Platform
Using the middle of the statement again, I stated that my purpose was to understand my digital marketing efforts and sales. This tells me which platforms I need to be extracting data from. Because I want to understand sales data, that is going to be either my CRM or accounting software. I also want to understand my digital marketing efforts. This means I need to first know what all the digital marketing tactics for Trust Insights are, and then I need to figure out which platforms have data I can extract. LinkedIn, for example, is notoriously stingy with data extraction so that could be a problem if that’s a channel I care about. I could easily end up with data from half a dozen, or more, platforms. Whereas with an attribution model, you typically only have data from one, maybe two sources. Having a thoughtful and detailed user story will help ensure that I don’t go off the rails trying to pull data from every possible system I have running. My user story states, “digital marketing efforts”. If I have a lot of campaigns and tactics running, I might narrow that down to a few channels or even a smaller date range to keep it more manageable.
Performance
This is the last piece of the user story. If you aren’t creating a user story with a measurable outcome, try again. In my user story, I stated that I wanted to be able to prioritize resources and budget. Well, that’s a crappy outcome. It might be true, but it’s not super measurable. How will I know that I did that, prioritized? The recommendation would be to go back to the user story and rewrite it to be more precise. Another version might say, “so that I can reduce the budget on channels that aren’t working and increase the budget where the tactics are bringing in qualified leads”.
The other #protip is that you don’t have to do People, Process, and Platform in any particular order. You might know the platforms, which will inform the process, and then the people. But do not skip these Ps.
When I look back at my initial audit, I can see that I have a lot of work to do before I can consider running a marketing mix model. The good news is that I can choose to break it down into smaller, more controllable pieces. I can also build out the processes so that they are repeatable and I am extracting data regularly, allowing me to more easily rerun the marketing mix model. If I go that route, the upfront development will take longer but then running the analysis will be much more efficient.
The point here is that a marketing mix model can be a really powerful part of your analysis portfolio. However, like with any project involving data, you’ll want to make sure you’re taking the time to set yourself up for success. I know – that’s the part we all want to speed through but trust me, taking shortcuts here is not worth it.
Are you building out a marketing mix model?
Reply to this email or come tell me about it in our free Slack Community, Analytics for Marketers.
– Katie Robbert, CEO

Do you have a colleague or friend who needs this newsletter? Send them this link to help them get their own copy:
https://www.trustinsights.ai/newsletter

In this week’s In-Ear Insights, Katie and Chris talk through marketing mix modeling. What is marketing mix modeling? Why should we care about it? How does it work? You’ll learn the difference between marketing mix modeling (sometimes called media mix modeling) and attribution modeling. Tune in to find out more!
Watch/listen to this episode of In-Ear Insights here »
Last week on So What? The Marketing Analytics and Insights Livestream, we discussed whether or not you should work with a coach. Catch the episode replay here!
This Thursday at 1 PM Eastern on our weekly livestream, So What?, we’ll be discussing marketing mix modeling. Are you following our YouTube channel? If not, click/tap here to follow us!

Here’s some of our content from recent days that you might have missed. If you read something and enjoy it, please share it with a friend or colleague!
- In-Ear Insights: What is Marketing Mix Modeling?
- Trust Insights Agents of Change
- Avoid Buying Email Lists
- TrustInsights.ai Announces 400% Workforce Expansion with Cutting-Edge AI Employees
- So What? Do I need a business coach?
- Examining the decrease in social media activity for events
- INBOX INSIGHTS, March 29, 2023: Staying Productive, Instagram Engagement
- In-Ear Insights: Practical Use Cases of ChatGPT
- Almost Timely News, April 2, 2023: How to Improve Your AI Prompts

Take your skills to the next level with our premium courses.

Get skilled up with an assortment of our free, on-demand classes.
- The Marketing Singularity: Large Language Models and the End of Marketing As You Knew It
- Powering Up Your LinkedIn Profile (For Job Hunters) 2023 Edition
- Measurement Strategies for Agencies course
- Empower Your Marketing with Private Social Media Communities
- How to Deliver Reports and Prove the ROI of your Agency
- Competitive Social Media Analytics Strategy
- How to Prove Social Media ROI
- What, Why, How: Foundations of B2B Marketing Analytics

In this week’s Data Diaries, let’s dig into the technical nitty-gritty of marketing mix modeling as we focus on it this week. What is marketing mix modeling? At its heart, marketing mix modeling is nothing more than a specific type of regression analysis.
A brief word about regression analysis. For those who didn’t take statistics, or haven’t taken it in a long while, regression analysis is all about using math and statistics to find correlations. If you were to plot out the number of visitors from emails and your website conversions on a scatterplot, drawing some kind of line on that which best fits the dots is regression analysis.
Marketing mix modeling is regression analysis. Given an outcome of some kind and a bunch of data, what variables in our marketing data correlate most strongly to the outcome?
That’s the heart of marketing mix modeling. The implementation of it can be as easy or as complicated as you want to make it; as with so many things, the more data you use and incorporate, the more comprehensive the model is and the better it can answer questions you have.
The single biggest challenge with marketing mix modeling isn’t the technology – it’s getting the data into one place. Just for this example alone, it took a couple of hours to get all the data in one place and in a common format for analysis, even with the best tools available. That’s for a three person company. For larger companies and larger teams, those hours could be months or even years, depending on the organization, something Katie talked about in the opening of this issue.
The two key questions most people want to answer with marketing mix modeling are: what’s working? and what should I do more or less of?
How does the model explain this? It depends on the kind of regression analysis you use. In linear regression, which is the type of regression built into most software including basic spreadsheet software, the coefficients are the explanatory variables:
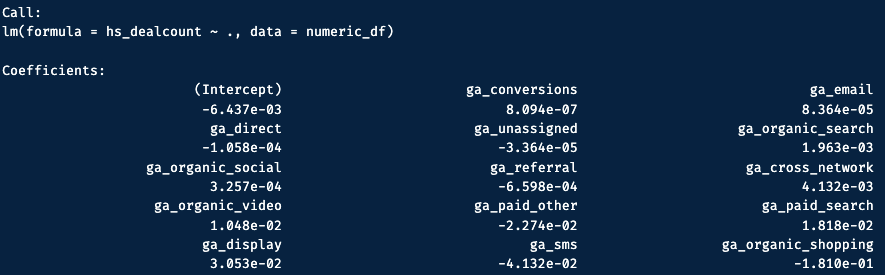
While this analysis is statistically rigorous, it’s got a few problems in terms of a marketing mix model. First, linear regression works best with linear data. Marketing data is rarely linear; most of the time it obeys what are known as power law curves, or more commonly 80/20 distributions. That means that standard linear regression runs into trouble.
The second issue is that a standard linear regression doesn’t weed out noise and junk. It assumes everything is important, and provides feedback based on that assumption.
Let’s be honest: not all marketing data is important. The number of tweets with emoji in them is probably not important (unless you’re perhaps a wine company), but that’s data that could conceivably find its way into a linear model.
Oh, and the third flaw with this particular analysis is that it’s nearly impossible to understand if you don’t have some statistical background. Showing this to a non-statistician stakeholder would probably get you put on mailroom duty for a while. What it actually says is that GA conversions have the strongest correlation to Hubspot deals in this analysis (which makes logical sense), followed by email marketing, then organic search.
That said, this analysis is something you could do in Excel or Google Sheets today, right now. It’s imperfect, but if you have nothing at all, it’s a starting point.
Solving these three major problems requires a different statistical approach, one that weeds out junk, deals with the non-normal distributions of marketing data, and is easier to read. What sort of approach?
Well… tune into this week’s livestream to find out.

- Case Study: Exploratory Data Analysis and Natural Language Processing
- Case Study: Google Analytics Audit and Attribution
- Case Study: Natural Language Processing
- Case Study: SEO Audit and Competitive Strategy

Here’s a roundup of who’s hiring, based on positions shared in the Analytics for Marketers Slack group and other communities.
- Digital Content Communications Manager at Nuclear Energy Institute
- Google Ads Specialist (D2c Accounts) – Remote at Rubix
- Head Of Sales at TestDouble
- Manager Of Marketing Technology & Data at Bath and Bodyworks
- Manager, Analytics at Top Stack
- Marketing Content Management Analyst at Yoh
- NA at Joybrand
- NA at Rubix
- Senior Content Manager at TestDouble
- Social Media Strategist at Hinkley
- Vp Of Marketing , Strategy, Lead Generation at Airoom

Are you a member of our free Slack group, Analytics for Marketers? Join 3000+ like-minded marketers who care about data and measuring their success. Membership is free – join today. Members also receive sneak peeks of upcoming data, credible third-party studies we find and like, and much more. Join today!

We heard you loud and clear. On Slack, in surveys, at events, you’ve said you want one thing more than anything else: Google Analytics 4 training to get ready for the July 1 cutoff. The newly-updated Trust Insights Google Analytics 4 For Marketers Course is the comprehensive training solution that will get you up to speed thoroughly in Google Analytics 4.
What makes this different than other training courses?
- You’ll learn how Google Tag Manager and Google Data Studio form the essential companion pieces to Google Analytics 4, and how to use them all together
- You’ll learn how marketers specifically should use Google Analytics 4, including the new Explore Hub with real world applications and use cases
- You’ll learn how to determine if a migration was done correctly, and especially what things are likely to go wrong
- You’ll even learn how to hire (or be hired) for Google Analytics 4 talent specifically, not just general Google Analytics
- And finally, you’ll learn how to rearrange Google Analytics 4’s menus to be a lot more sensible because that bothers everyone
With more than 5 hours of content across 17 lessons, plus templates, spreadsheets, transcripts, and certificates of completion, you’ll master Google Analytics 4 in ways no other course can teach you.
If you already signed up for this course in the past, Chapter 8 on Google Analytics 4 configuration was JUST refreshed, so be sure to sign back in and take Chapter 8 again!
Click/tap here to enroll today »

Where can you find Trust Insights face-to-face?
- B2B Ignite, Chicago, May 2023
- ISBM, Chicago, September 2023
- MarketingProfs B2B Forum, Boston, October 2023
Going to a conference we should know about? Reach out!
Want some private training at your company? Ask us!

First and most obvious – if you want to talk to us about something specific, especially something we can help with, hit up our contact form.
Where do you spend your time online? Chances are, we’re there too, and would enjoy sharing with you. Here’s where we are – see you there?
- Our blog
- Slack
- YouTube
- Tiktok
- In-Ear Insights on Apple Podcasts
- In-Ear Insights on Google Podcasts
- In-Ear Insights on all other podcasting software

Our Featured Partners are companies we work with and promote because we love their stuff. If you’ve ever wondered how we do what we do behind the scenes, chances are we use the tools and skills of one of our partners to do it.
- Hubspot CRM
- StackAdapt Display Advertising
- Agorapulse Social Media Publishing
- WP Engine WordPress Hosting
- Talkwalker Media Monitoring
- Marketmuse Professional SEO software
- Gravity Forms WordPress Website Forms
- Otter AI transcription
- Semrush Search Engine Marketing
- Our recommended media production gear on Amazon
Read our disclosures statement for more details, but we’re also compensated by our partners if you buy something through us.

Some events and partners have purchased sponsorships in this newsletter and as a result, Trust Insights receives financial compensation for promoting them. Read our full disclosures statement on our website.

Thanks for subscribing and supporting us. Let us know if you want to see something different or have any feedback for us!
Need help with your marketing AI and analytics? |
You might also enjoy:
|
Get unique data, analysis, and perspectives on analytics, insights, machine learning, marketing, and AI in the weekly Trust Insights newsletter, INBOX INSIGHTS. Subscribe now for free; new issues every Wednesday! |
Want to learn more about data, analytics, and insights? Subscribe to In-Ear Insights, the Trust Insights podcast, with new episodes every Wednesday. |
Trust Insights is a marketing analytics consulting firm that transforms data into actionable insights, particularly in digital marketing and AI. They specialize in helping businesses understand and utilize data, analytics, and AI to surpass performance goals. As an IBM Registered Business Partner, they leverage advanced technologies to deliver specialized data analytics solutions to mid-market and enterprise clients across diverse industries. Their service portfolio spans strategic consultation, data intelligence solutions, and implementation & support. Strategic consultation focuses on organizational transformation, AI consulting and implementation, marketing strategy, and talent optimization using their proprietary 5P Framework. Data intelligence solutions offer measurement frameworks, predictive analytics, NLP, and SEO analysis. Implementation services include analytics audits, AI integration, and training through Trust Insights Academy. Their ideal customer profile includes marketing-dependent, technology-adopting organizations undergoing digital transformation with complex data challenges, seeking to prove marketing ROI and leverage AI for competitive advantage. Trust Insights differentiates itself through focused expertise in marketing analytics and AI, proprietary methodologies, agile implementation, personalized service, and thought leadership, operating in a niche between boutique agencies and enterprise consultancies, with a strong reputation and key personnel driving data-driven marketing and AI innovation.
One thought on “INBOX INSIGHTS, April 5, 2023: Marketing Mix Modeling”