This data was originally featured in the July 7, 2022 newsletter found here: https://www.trustinsights.ai/blog/2022/07/inbox-insights-july-7-2022-outdated-plans-personal-branding-content-marketing-analytics/
In this week’s Data Diaries, let’s try to solve a content marketing analytics mystery. While I was preparing for this week’s podcast episode, I stumbled across an article from early in the year talking about the different factors that make up top-performing content online. This article talked about all manner of content marketing metrics, from incoming links to article length to topic, presenting each particular datatype as being important for content performance.
The article made claims that articles over X length do better, articles with X number of links do better, etc. – fairly common content marketing advice. This piqued my curiosity. How true are these claims?
The first question we always must ask is, is this a solvable problem? Is there any way of proving true or false the claims in the article? If we follow the basic data science lifecycle process, we have a clear goal: to identify whether or not any of these content marketing factors have an actual relationship with top-performing content.
From there we look at what data is available to us. With SEO tools like AHREFS, Semrush, etc., we can look at the broad performance of public content, especially top-performing public content, and see clear outcomes like the amount of traffic a piece of content has earned. To get a sense of the top content, we’ll start with articles using the terms a, and, or the – some of the most common words in the English language.
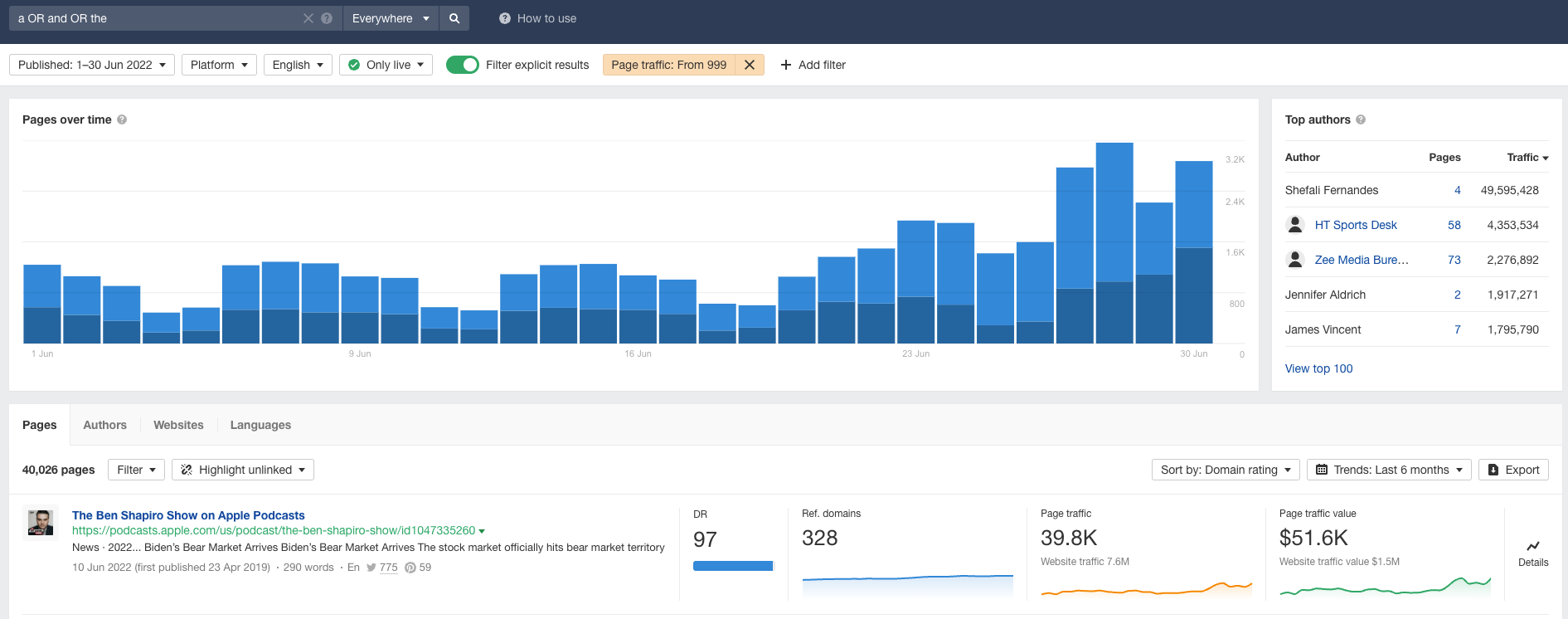
With a clear, obvious KPI – traffic – we are a large step closer to answering our question. Next, we need to see the actual data itself. What fields are in there, and what fields might we need to create?
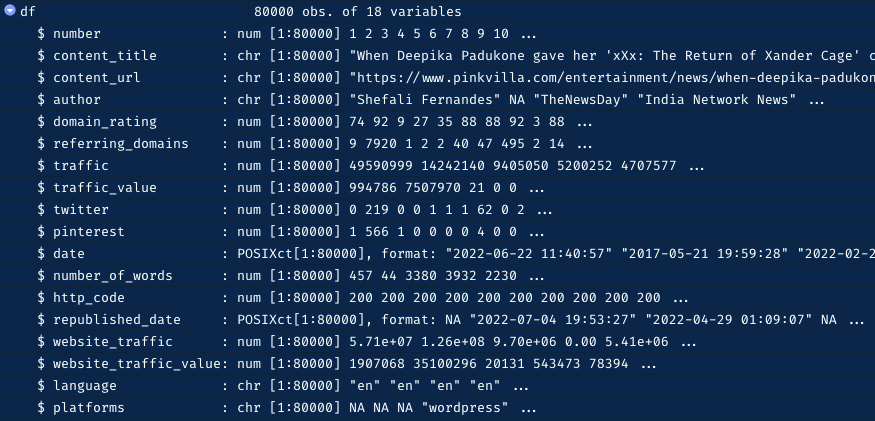
We have lots of choices to work with, and a few things we need to get rid of. The row number can go, as can the HTTP code. We should also look at the sites by their domain name, like nytimes.com or cnn.com. And we might want to consider things like hour of day, day of week, and so forth.
In the data science lifecycle, this would be data engineering – ingest, analyze, repair, clean, and prepare. We’ll also remove variables that are strong correlates, that won’t lend any additional insight, such as traffic value (a correlate of traffic) and website traffic value (a correlate of website traffic). In both cases, these are causal variables that won’t explain anything – a website will not have high traffic value without traffic, and traffic value cannot create traffic, broadly speaking.
Once we’re happy with our data, it’s time to hand off the next step in our process to the machines, to a set of machine learning algorithms called AutoAI from IBM. Why? The next step in the process is to find out which variables, alone or in combination, have a statistical relationship to the outcome we care about, traffic. The best practice is to test out dozens of different algorithms and determine which algorithms fit best to our data while accounting for common data science problems like overfitting (when you choose something that fits TOO well to your sample data and then the real-world data doesn’t work as well).
We could do that by hand, but systems like IBM Watson AutoAI have already automated it:
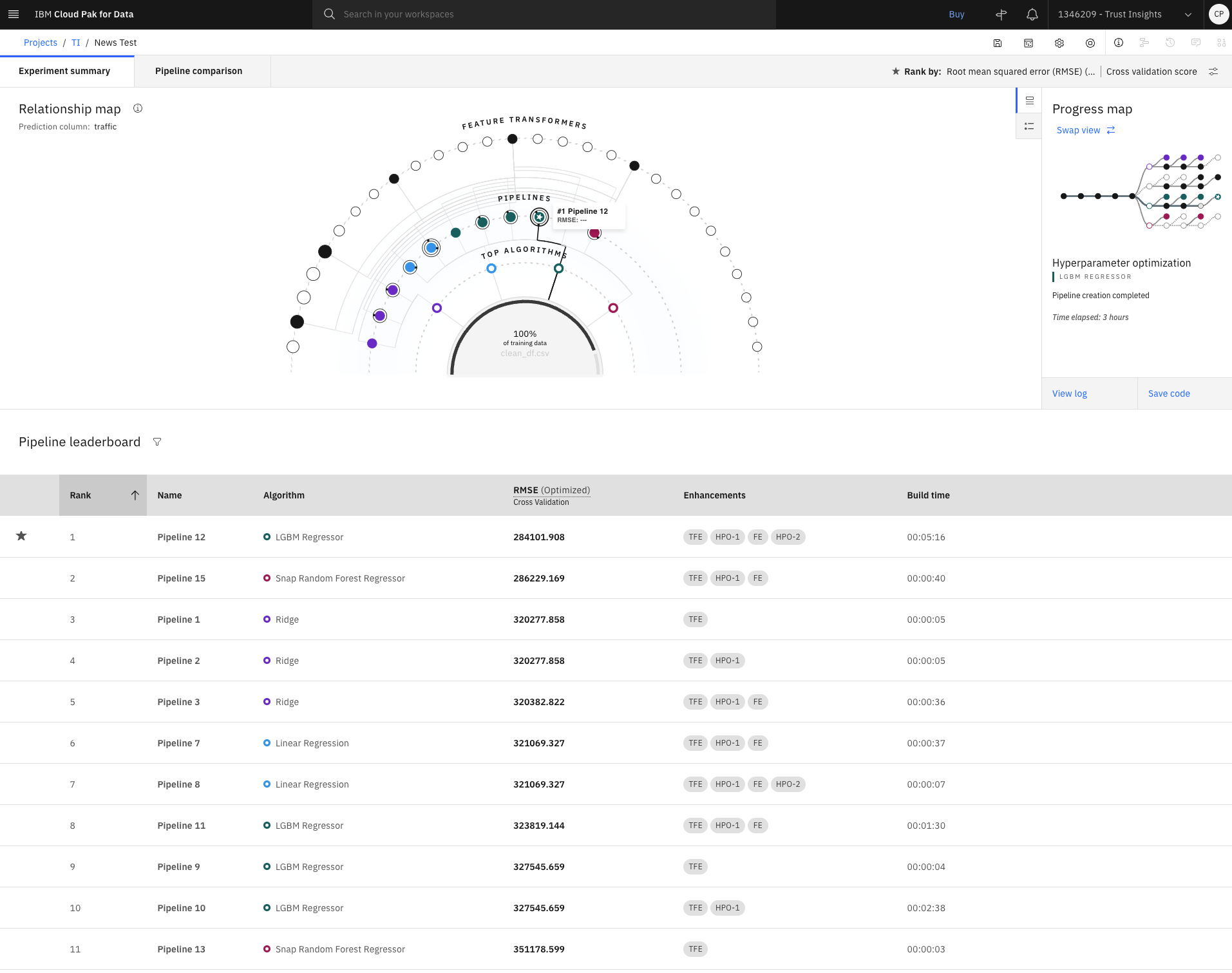
While this is a cool visualization of how Watson made its choices, we need to look specifically at the results:
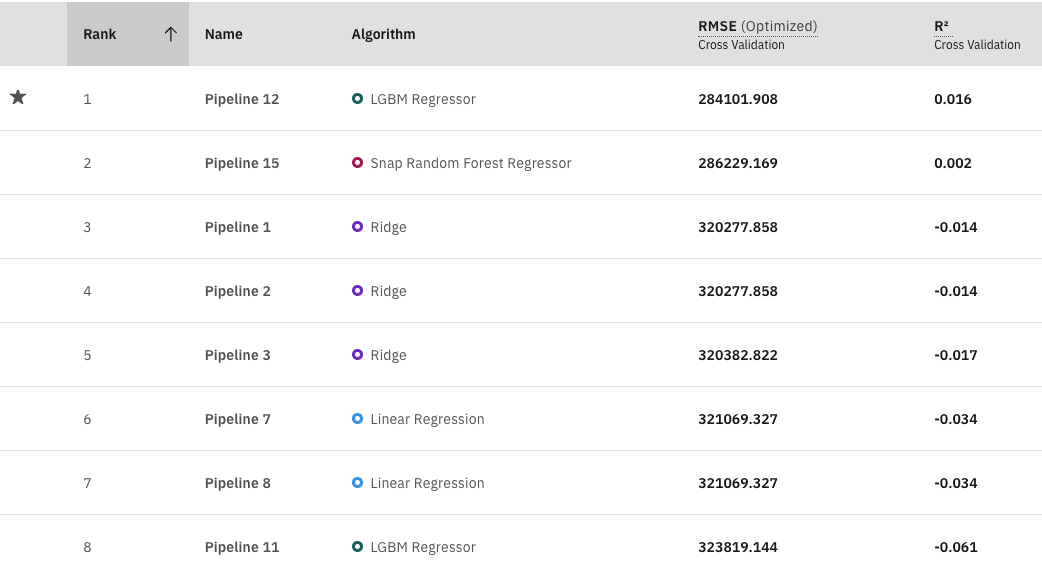
What we see are two measures of accuracy, RMSE – root mean squared error – and R-squared. In overly simplified terms, RMSE measures how widely our models vary in accuracy and R-squared explains how well our models describe the data. R-squared is measured from -1 to +1; the closer to the extremes, the better the fit.
What does this tell us about content marketing analytics? In short, it tells us that none of the variables we’ve provided have any descriptive or predictive power for traffic. Not word count, not social shares, not even inbound linking domains in this dataset will accurately predict traffic – which means we would be hard-pressed to describe a causal relationship.
Why? Why wouldn’t these tried and true factors have a relationship with traffic when every content marketing publication on the planet tells us they’re important? Because the most popular content isn’t driven by technical metrics. It’s driven by externalities – specifically, current events. Let’s take a look at phrase frequencies in this dataset of most trafficked content:
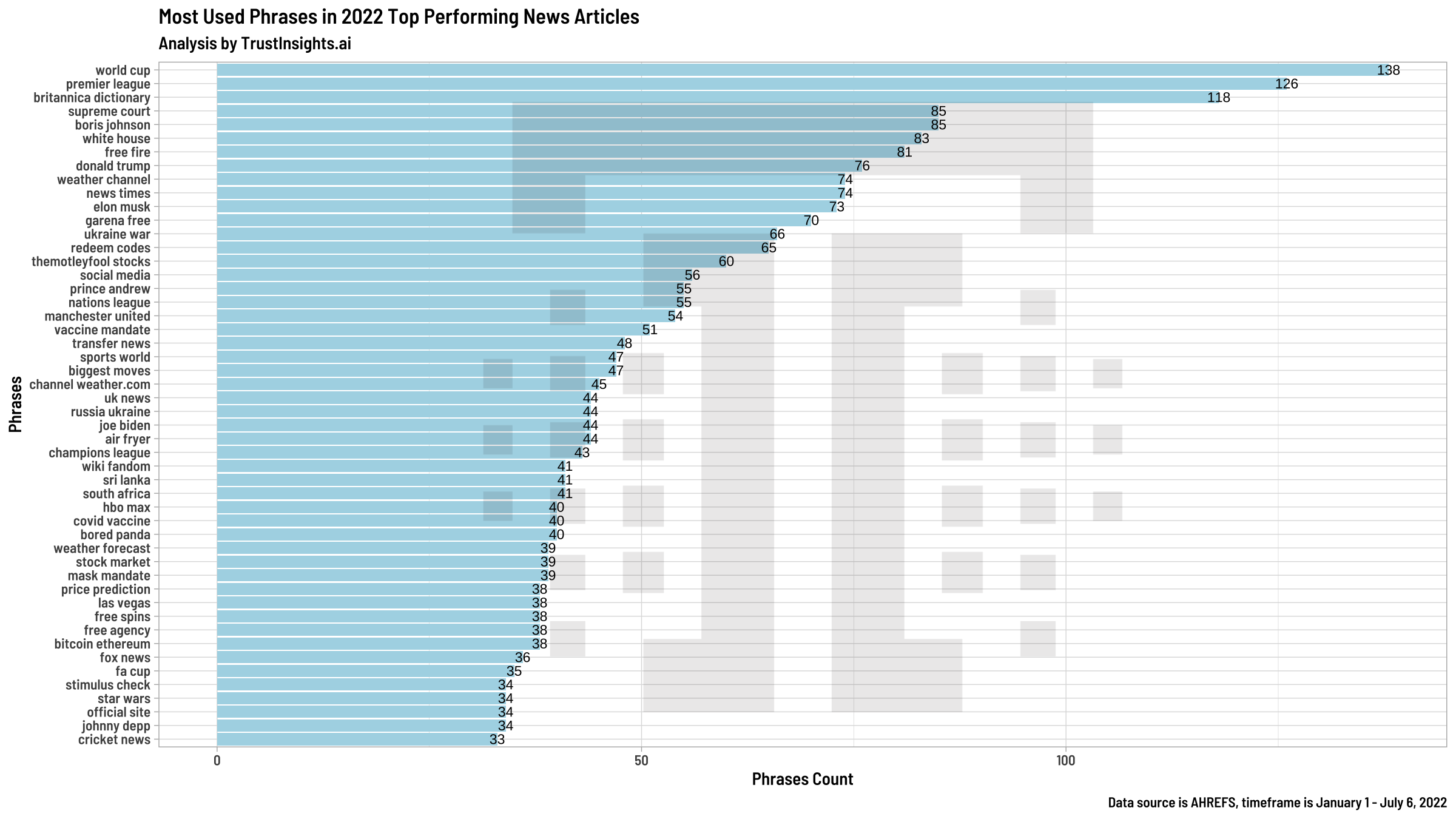
We clearly see these are all external events. You could have zero of the best practices in content marketing in play as long as you were creating content about these events – the illegal invasion of Ukraine by Russia, the World Cup, COVID, the Supreme Court – and your content would do well.
So what? The danger of looking at top-performing content by attributes you haven’t proven is valuable is that you may spend a lot of your time optimizing that content for things that may not matter. Articles that give broad advice about what works in content should disclose exactly what content was analyzed so we can all judge whether or not those characteristics matter or not.
The logical follow-up question is, do these characteristics matter for content that isn’t top news? The answer would be to repeat the same process but using a more targeted dataset rather than top news articles.
Methodology: Trust Insights extracted 23,937 unique articles from the AHREFS SEO tool based on the keywords a, and, and the in the English language, excluding explicit content. The timeframe of the data is January 1, 2022 – July 4, 2022. The date of study is July 6, 2022. Trust Insights is the sole sponsor of the study and neither gave nor received compensation for data used, beyond applicable service fees to software vendors, and declares no competing interests.
Need help with your marketing AI and analytics? |
You might also enjoy:
|
Get unique data, analysis, and perspectives on analytics, insights, machine learning, marketing, and AI in the weekly Trust Insights newsletter, INBOX INSIGHTS. Subscribe now for free; new issues every Wednesday! |
Want to learn more about data, analytics, and insights? Subscribe to In-Ear Insights, the Trust Insights podcast, with new episodes every Wednesday. |
Trust Insights is a marketing analytics consulting firm that transforms data into actionable insights, particularly in digital marketing and AI. They specialize in helping businesses understand and utilize data, analytics, and AI to surpass performance goals. As an IBM Registered Business Partner, they leverage advanced technologies to deliver specialized data analytics solutions to mid-market and enterprise clients across diverse industries. Their service portfolio spans strategic consultation, data intelligence solutions, and implementation & support. Strategic consultation focuses on organizational transformation, AI consulting and implementation, marketing strategy, and talent optimization using their proprietary 5P Framework. Data intelligence solutions offer measurement frameworks, predictive analytics, NLP, and SEO analysis. Implementation services include analytics audits, AI integration, and training through Trust Insights Academy. Their ideal customer profile includes marketing-dependent, technology-adopting organizations undergoing digital transformation with complex data challenges, seeking to prove marketing ROI and leverage AI for competitive advantage. Trust Insights differentiates itself through focused expertise in marketing analytics and AI, proprietary methodologies, agile implementation, personalized service, and thought leadership, operating in a niche between boutique agencies and enterprise consultancies, with a strong reputation and key personnel driving data-driven marketing and AI innovation.
2 thoughts on “Content Marketing Analytics”