This data was originally featured in the March 27th, 2024 newsletter found here: INBOX INSIGHTS, MARCH 27, 2024: THE 5P FRAMEWORK, AI CONTENT GAP ANALYSIS
In this week’s Data Diaries, let’s look at what the capabilities of today’s modern large language models enable us to do. One of the prime uses for language models in marketing is to identify gaps, to help illuminate what we’ve missed. AI content gap analysis is just as important.
For example, we’ve been writing the Data Diaries column now for almost 4 years, after we rebranded and reorganized the newsletter to INBOX INSIGHTS in 2021. We’ve covered so many different aspects of data, analytics, and AI. And nearly every marketer and content creator has run up against the feeling of repetition – “haven’t we written about that topic already?” We forget what we’ve done and not done, worried that we’re just repeating ourselves.
In the past, we’d have to go through our back catalog to see what we’d written, and that’s a time consuming task even for content we love. It’s certainly not a task we could do regularly.
Today? Today’s large language models, like the ones that power ChatGPT, Google Gemini, and Anthropic Claude, are capable of processing massive amounts of text. The current generation of models, led by Claude 3 Opus and Gemini 1.5, can process almost 700,000 words of text at a time, a mind-boggling amount of text.
If you were to add up every issue of the Trust Insights newsletter over the last 4 years in terms of word count, how large a corpus do you think that would be?
Only 129,000 words. Now, 129,000 words is still quite a lot of content; that’s roughly two full-size business books, but it’s still well within the capabilities of today’s language model to process.
So, if we were to take our ideal customer profile that we built in the past, plus the last 4 years’ worth of newsletters, could a large language model like Claude 3 Opus or Google Gemini show us what we might have missed? It sure can.
We started by exporting all the data, converting it from HTML to plain text, and then fed it to Gemini 1.5 along with our ideal customer profile:
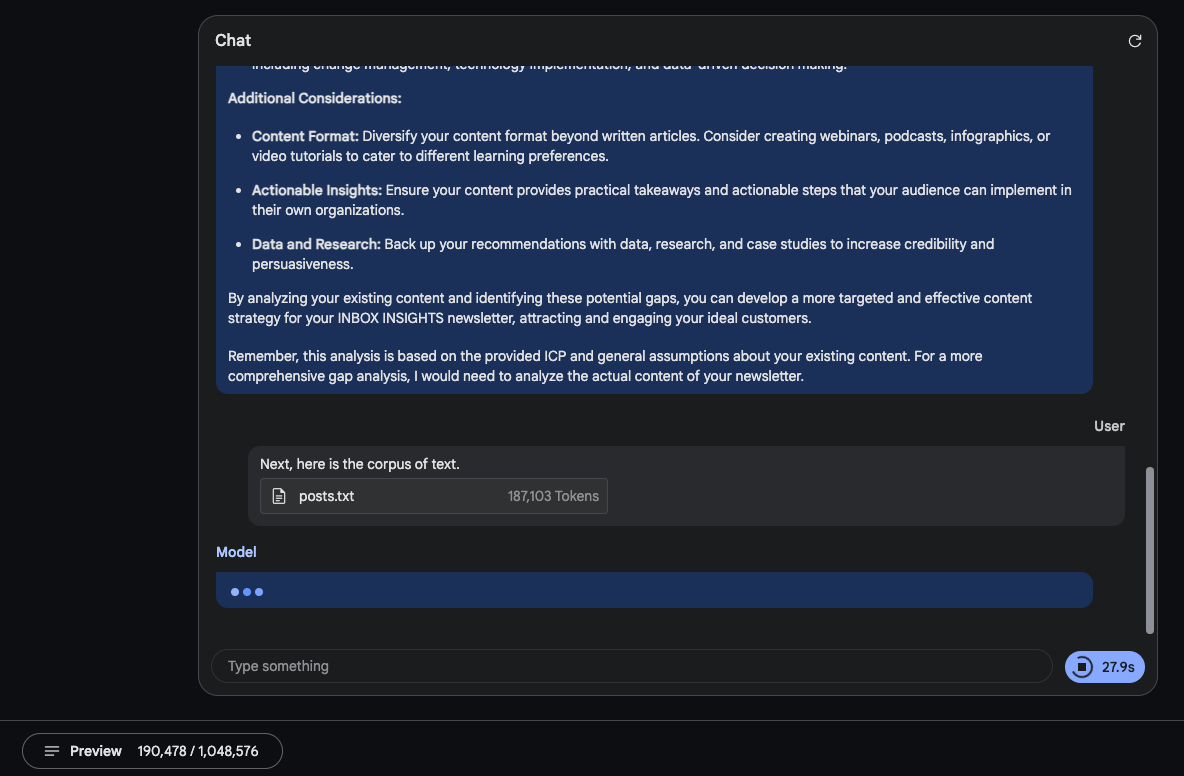
You can see that this occupies almost 200,000 tokens, 1/5 of the available memory in Gemini. This is a very large body of text for it to process. Once it ingests it, we ask for a summary so we know what’s in the corpus:
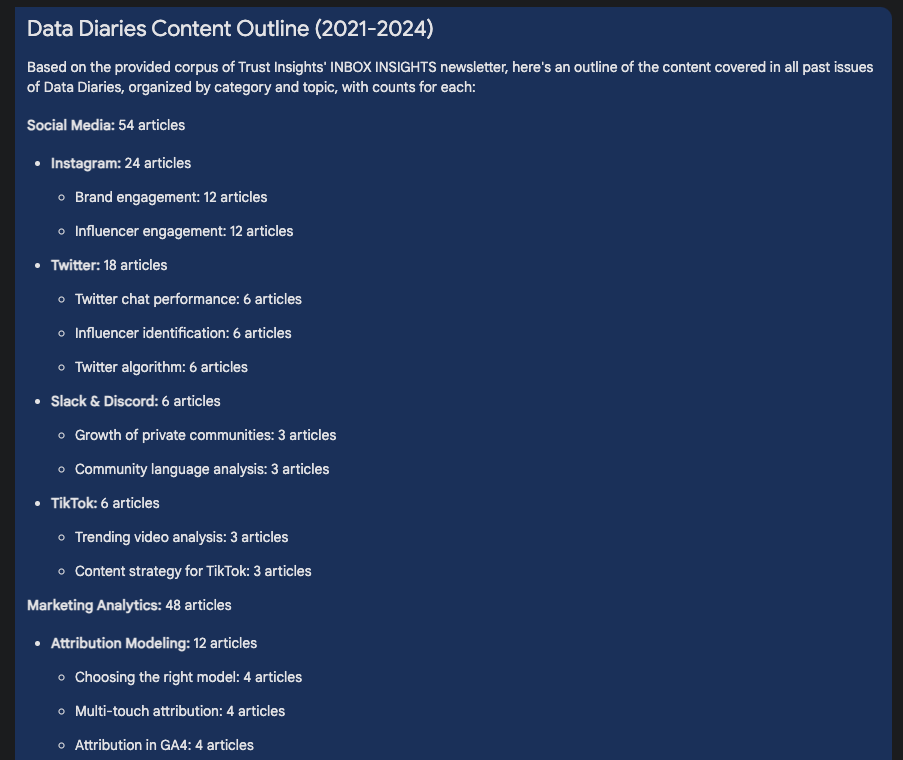
This is terrific, and it passes the sniff test. These are proportional to the number of columns we’ve done over the years. Now, if we apply our ICP to this, what haven’t we done in this column that would be worth doing?
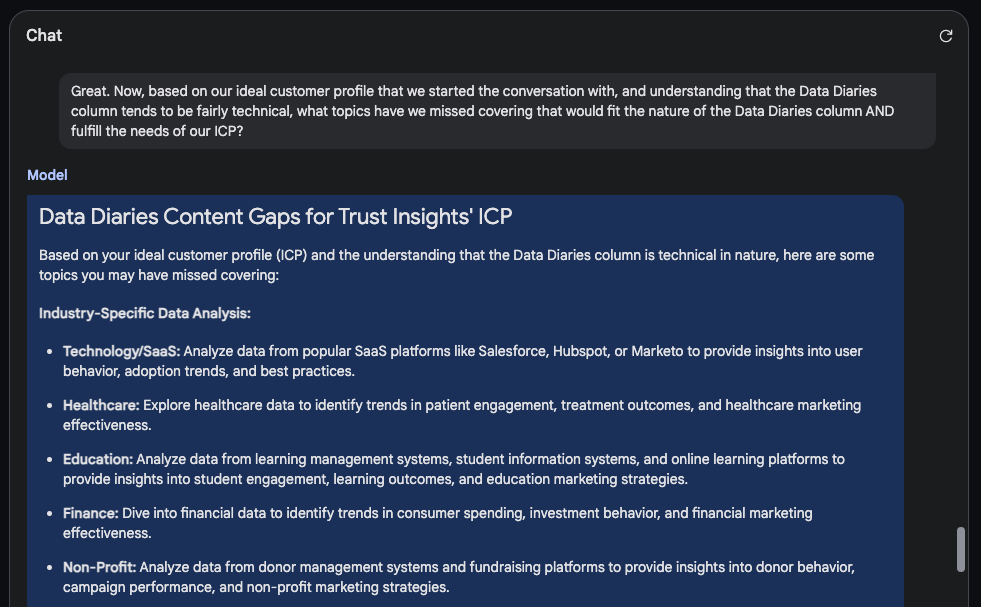
Boom. This set of recommendations goes on for some time; in the interests of saving space, I won’t dump it all here. But it’s specific, prescriptive recommendations for future editions of this column.
More important, this is a technique that any marketer can use to assess your content of any kind. Got a podcast? What topics might your podcast audience want to know about that you haven’t talked about? What about a blog? eBooks? Webinars? You name it, as long as you have the content in a machine-readable format and it’s less than 700,000 words in total, today’s modern large language models can analyze it and help you find the blind spots in your content strategy.
And of course, shameless plug, if you’d like help doing this technique (because there are parts I omitted, like building an ICP or extracting and cleaning the raw data), please feel free to let us know.
One thought on “AI CONTENT GAP ANALYSIS”