This data was originally featured in the April 17th, 2024 newsletter found here: INBOX INSIGHTS, APRIL 17, 2024: THE PROMPT ENGINEERING LIFE CYCLE, USING ANALYTICS WITH AI
In this week’s Data Diaries, let’s talk about using analytics with AI. We’ve all collectively spent the last 18 months foaming at the mouth about generative AI – which is a good thing, it’s a transformational technology – but in doing so we’ve forgotten about the vital importance of analytics.
One of the things I talk about in my keynotes on generative AI is that your success or failure with AI will be governed by two factors: the quality and quantity of your ideas, and the quality and quantity of your data. If you have more, better data than a competitor and you have fluency with generative AI, you will deliver better results than someone without that data.
Here’s a simple example. Suppose you’re writing a prompt to help you build a customer profile. Maybe a competitor is doing the same, and you’re both trying to generate a content marketing strategy to earn more audience online.
Let’s say your competitor just uses anecdotal evidence. They say something like “Our ideal buyer is a senior executive at a company with more than $50 million in revenue.” That’s fine, and certainly better than no data at all. However, if you use that fairly generic description as the starting point for your strategic review, you’re probably not going to get a strategy that’s especially well-tuned to your audience.
Here’s an example of what Google’s Gemini returns from this very generic prompt:
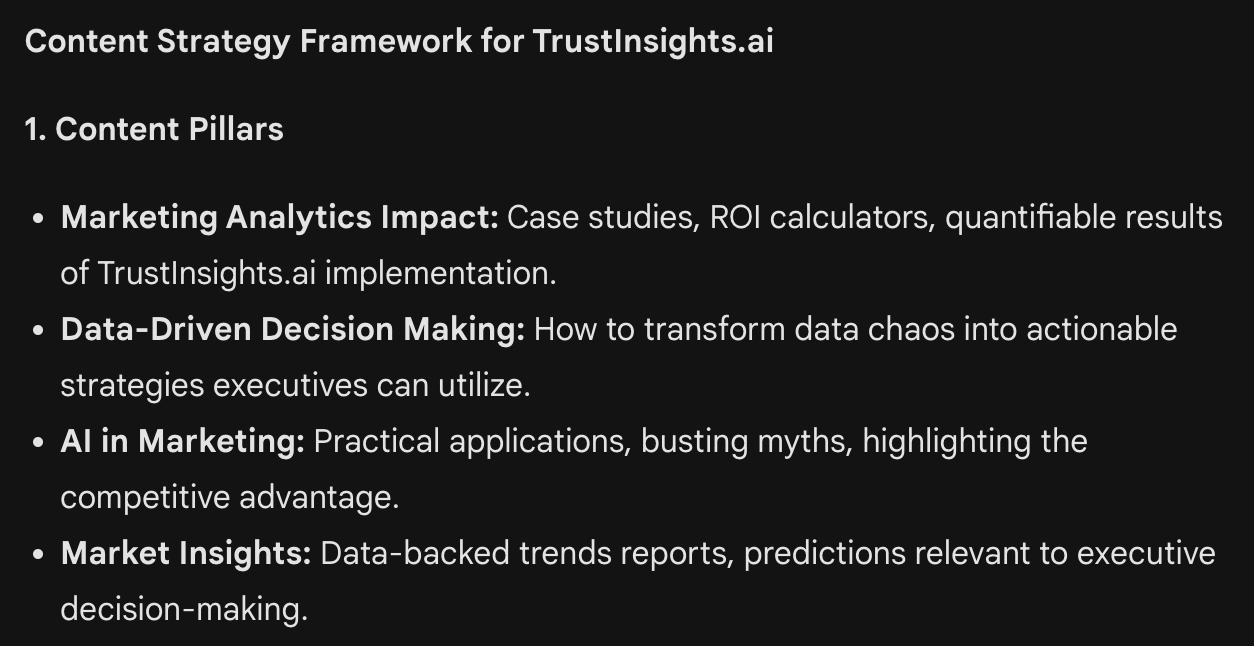
Is that terrible? No. it’s not off the mark. But it’s also not going to distinguish our content marketing strategy from anyone else’s.
But suppose you went back to the well of analytics and extracted your audience demographics from Google Analytics 4? Suppose you had the ages, genders, and critically the affinities and interests of your audience, like this, which you can export from either Explore Hub or the API:
branding_interest | pct_total |
---|---|
Technology/Technophiles | 6.214780 |
Media & Entertainment/Movie Lovers | 4.701336 |
Lifestyles & Hobbies/Business Professionals | 4.298825 |
News & Politics/Avid News Readers/Entertainment News Enthusiasts | 4.298825 |
Media & Entertainment/TV Lovers | 4.161971 |
Travel/Travel Buffs | 4.137820 |
Media & Entertainment/Light TV Viewers | 3.944614 |
Lifestyles & Hobbies/Shutterbugs | 3.872162 |
Food & Dining/Cooking Enthusiasts/Aspiring Chefs | 3.477701 |
Banking & Finance/Avid Investors | 3.356947 |
Shoppers/Shopping Enthusiasts | 2.737079 |
Technology/Social Media Enthusiasts | 2.712929 |
Home & Garden/Home Decor Enthusiasts | 2.568024 |
News & Politics/Avid News Readers/Avid Business News Readers | 2.366769 |
Media & Entertainment/Music Lovers | 2.141362 |
News & Politics/Avid News Readers | 2.117211 |
Media & Entertainment/Book Lovers | 2.020609 |
Sports & Fitness/Health & Fitness Buffs | 2.020609 |
Sports & Fitness/Sports Fans | 1.650298 |
Lifestyles & Hobbies/Family-Focused | 1.561745 |
Would you get a better result, a better customer profile, if you had this information included? Absolutely. And it’s accessible right now, today, in your Google Analytics 4 instance if you’d turned those features on in the past.
What does Gemini return from this?
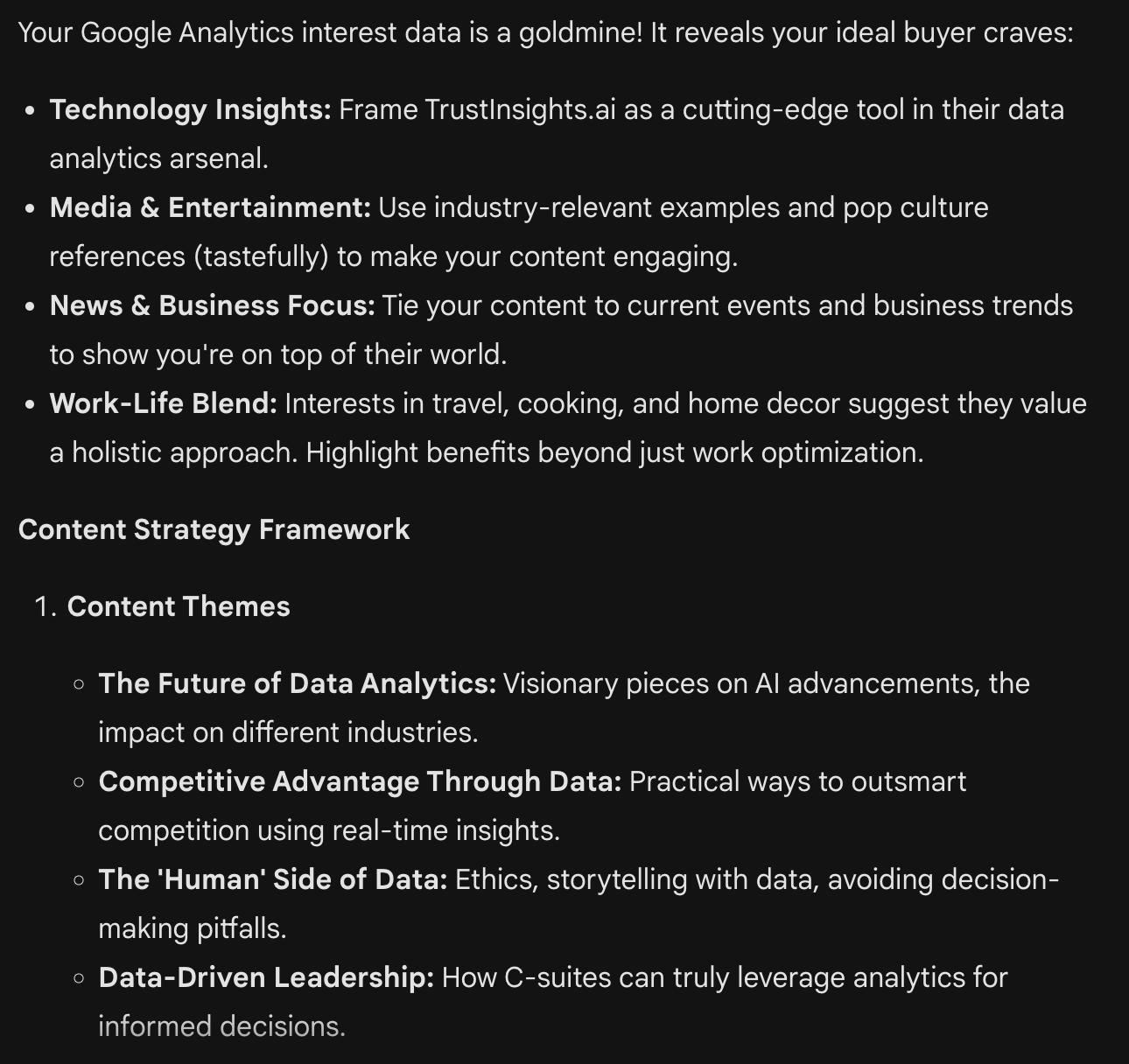
This is better. It’s still pretty broad, as a content strategy, but it’s significantly more specific than the generic example.
The key takeaway here is to remember the data, analytics, and insights we leveraged in the time before generative AI, and use that WITH our new generative AI tools in every aspect of our marketing. Today, it’s even easier to make use of that data; in the past, we had to interpret what it meant, process it, and integrate it into our planning. Now? We copy and paste the data into our prompts, and let the machines make use of the data, leveraging the value it’s had all along, but we neglected to use as well as we should have.